1. Poor or Insufficient Training Data
- Training data that doesn’t cover enough real-world scenarios the model may fail to generalize well and handle all real-world input.
- Training data that is noisy or contains errors, which can easily happen with data scraped from the broad internet, then the results will be unreliable.
Models that evolve over time can become outdated if the training data isn’t constantly updated with validated, reliable training data.
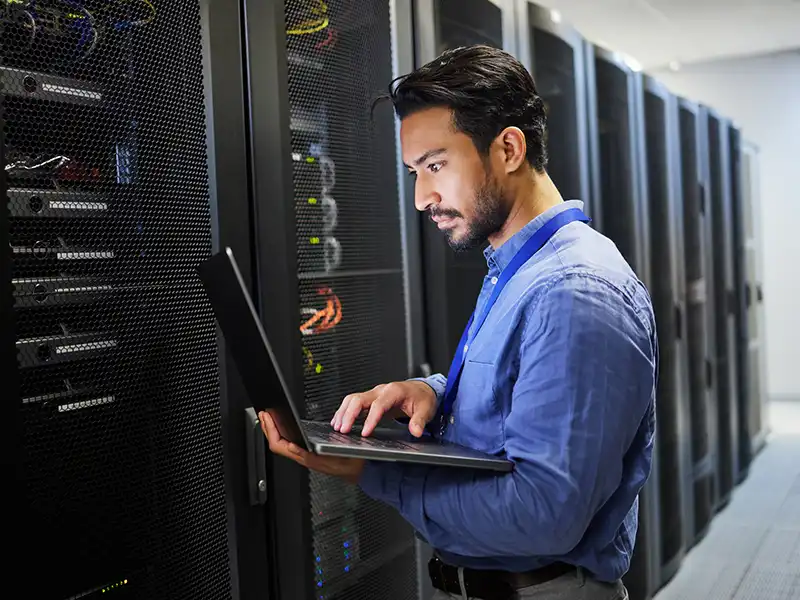
2. Data Bias
Representation and amplification bias are all potential bias risks that can inadvertently be built into models
Representation bias happens when a particular group built into the model isn’t representative of the overall targeted population
Amplification bias can exaggerate subtle biases in the data if the training process overemphasizes those patterns.
3. Model Robustness
Models may fail in unexpected conditions or produce errors, not because of data, but because of model architecture, training process, or ability to handle inputs.
This contrasts with training and bias which originate directly from the training data.
The risks associated with model robustness can include liability for harmful or illegal output or potential intellectual property violations if the model leaks proprietary or confidential data.
4. Scalability and Performance
- Scaling can cause slow performance and high resource use that degrades user experience and increases costs.
- A model may work in a controlled environment but that does not mean it is ready to handle demand growth, input complexity or real-world applications.
Scaling growth and real-world applications have the potential to increase computational resources, latency times, throughput, energy consumption and response times for the user.
5. Data Privacy and Breaches
- Data privacy and breach risks result from the handling, storage, and potential exposure of sensitive information used to train, fine-tune, or interact with generative AI models.
- Models often rely on huge datasets that may include personal, proprietary, or confidential data.
- Users interacting with generative AI may input sensitive information (e.g., ePHI, business plans) in prompts. If the system logs or reuses this data without consent—e.g., for further training—it risks breaching confidentiality
- Laws like GDPR , CCPA , or HIPAA impose strict rules on handling personal data. Generative AI’s data pipelines can violate these regulations if PII isn’t anonymized, or consent isn’t obtained.
6. Model Theft and Reverse Engineering
Model theft happen if an unauthorized user gained access to a model’s components such as weights, architecture, training data through hacking, insider leaks. This intellectual property could then be used or sold.
Reverse engineering involves analyzing a model’s output, behavior, or API interactions to infer its design, parameters, or training data
Researchers from Google published a paper in March 2024 title “Stealing Part of Production Language Model” that presented a model-stealing attack targeting production LLM’s like ChatGPT.
The risks involve intellectual property theft, data privacy violations, contract breaches all with legal, financial, and reputation implications for a company.
7. Ethics and Transparency
- Generative AI can produce convincing but false content, misleading users or amplifying misinformation.
- Models training on biased datasets can perpetuate stereotypes, discrimination, or unfair outcomes, even unintentionally.
- Training data that contains personal or copyrighted material scraped without explicit consent raises ethical questions about ownership and privacy.
- Companies may not reveal use of AI-generated content deceiving users about it origins.
- All of these can lead can lead to misinformation and copyright lawsuits or regulatory compliance risk.
8. Adversarial Attacks
- Adversarial attacks involve manipulating inputs such as text prompts, images, or other data to exploit vulnerabilities in the model, causing it to produce incorrect, unintended or harmful outputs. For example, in 2023, users discovered “jailbreak” prompts to bypass ChatGPT’s safety filters.
- here are multiple approaches to adversarial attacks including input perturbation, Tgradient-based attacks, prompt engineering exploits, transferability, and output manipulation.
- These attacks can expose generative AI companies to legal issues such as intellectual property violations, data privacy breaches in violation of GDPR or CCPA, and harmful content liability. It also poses regulatory non-compliance risks with the EU AI Act, HIPAA, or PCI-DSS.
9. Third-Party Risk
- All of the same third-party supply chain risks that companies manage today exist when dealing with generative AI platforms.
- Third-party data providers may supply training datasets which may be incomplete, biased, or contain errors.
- Third-parties handling sensitive data such as cloud storage or other aspects of the Generative AI platform may suffer breaches.
- Third-parties such as contractors or open-source contributors may leak or misuse proprietary algorithms, weights, or training data eroding competitive advantage.
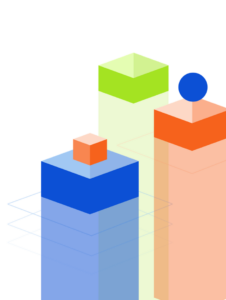
10. Lack of Governance
All of these risks make it clear that boards and leadership should establish formal governance over generative AI projects to avoid potential financial, reputational, intelectural property, legla, and regulatory risks
Establish a clear “Artificial Intelligence Acceptable Use Policy” so that employees don’t misuse AI or mistakenly divulge confidential information to 3rd party AI agents or systems.